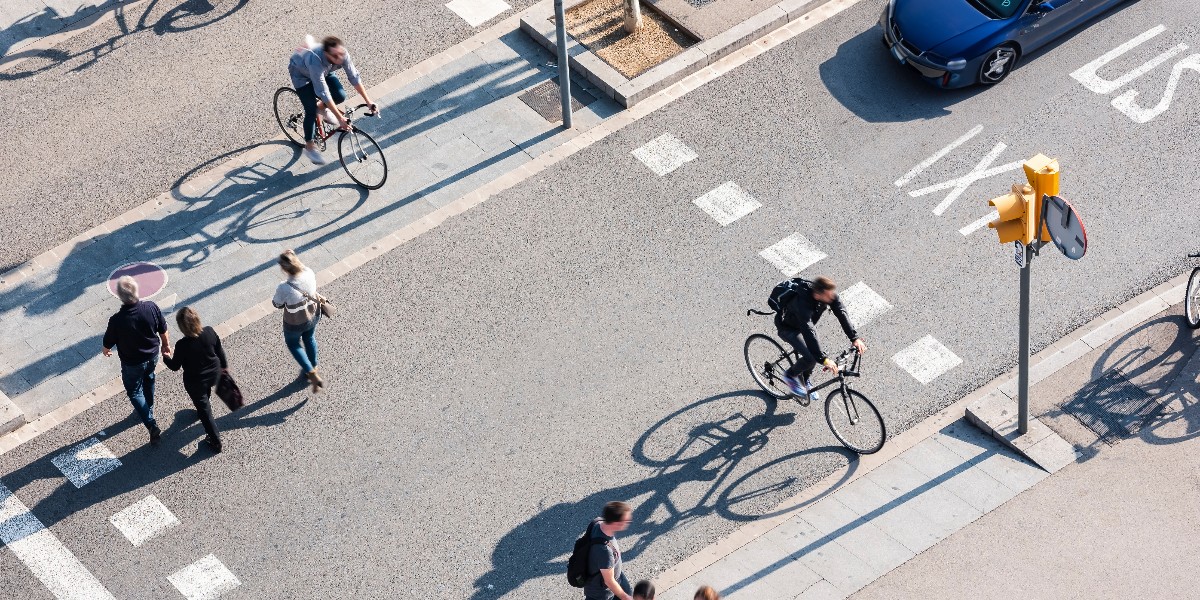
Anders Johansson, CTO and co-founder of CrowdVision (acquired by Skyfii), presented at the British Chamber of Commerce in Hong Kong Greater Bay Area Summit 2021 on how to harness data to build a smart city. Anders shares his insights from this presentation.
Smart cities have a few competing perspectives when it comes to how they should start. Whether the decision be a top-down or bottom-up approach, technology or community-led, or purposefully designed or naturally evolving, etc.
It’s important to include a little bit of all of the above.
In order to optimise cities and inform decision making, data is required. In order to collect data, you need an appropriate digital backbone with sensors and high-bandwidth low-latency connectivity.
Governments and city authorities are in a good position to plan and execute on infrastructure projects. They can provision not only physical infrastructure but also the digital backbone needed to make a city smart (e.g. 5G connectivity, various types of sensing technologies, etc).
However, governments are not necessarily the best suited to provide innovative services using this digital infrastructure to improve the lives of citizens.
Therefore, many cities around the world make their real time and historic datasets available to the public through open APIs. This allows an ecosystem of entrepreneurs, academics, start-ups, and tech companies to innovate and provide novel services utilising those datasets.
In this way, a citizen may be a consumer of services, but also a producer of new services, and a data point in some of the datasets.
Citizen Decision Making
In cities with a healthy ecosystem of openly available data feeds and services built upon those, citizens have the tools to help them navigate cities, seek out opportunities, etc. Also, citizens gradually learn from their previous decisions and hone their skills to become ‘street smart’ by the use of simple heuristics (rules of thumb) to guide them in their daily decision making.
Government Decision Making
In order to plan and design for the future, governments use much of the same datasets to inform their own decisions and policies.
However, there is a conundrum— should a government assess the demand for a service or utility and then design to meet that demand (e.g. if the roads are congested, should they build more roads?), or should they have a vision of what they want their future city to be (e.g. carbon neutral) and use policies to affect citizens to gradually take them to that intended outcome (e.g. discourage the use of petrol cars)?
A bit of both is needed since cities need to function properly in the interim until a future vision of the city can be realised.
Unlike for citizens though, when the government makes a wrong decision, the costs can be high. So, it makes sense to invest time and money into collecting the necessary data and building models to test potential scenarios, hypothetical policies, infrastructure investments, etc.
Modelling
A common way to address these challenges is to build a digital twin. A digital twin is a digital replica of a physical space like an airport or even a whole city, where you can test the impact of different policies or other decisions before they are implemented in the real world.
Digital twin modelling requires not only data but also sophisticated models. These models need theories of human behaviour to support them.
Even though there is an abundance of data to analyse and mine, it’s still important to build a model. If decisions are not supported by quality data, sound theory, and models, there is a risk that well-intended infrastructure projects and policies make the situation even worse than what it was before.
An example of this is Braess’s paradox which shows the transport network or electric grid capacity can sometimes be worse off by building a new road or by adding a new link. This may seem counterintuitive but there are many real-world examples of when this phenomenon has occurred.
So, what kind of models help avoid these kinds of issues?
Agent Based Modelling is an option and includes a set of interacting virtual humans (or ‘agents’) that lead to emergent outcomes at the macro scale.
Alternatively, Causal AI can help. This modelling differs from traditional machine learning in the sense that the causal relationships in the data are identified rather than just correlations in the data. This greatly reduces the risk of policies or decisions going wrong.
How does this relate to smart cities?
All data that currently exists reflects behaviour during a specific set of conditions and circumstances. That’s why it’s important to identify the cause and effect in possible smart city scenarios to avoid the risk of the unfortunate outcomes mentioned above.
In a well-functioning city, technology should augment and guide citizens in subtle ways, without having an overly intrusive impact on their lives.
Learn more about the benefits of smart cities and how we can help create a smart city solution for your city.